By Vijaya Joshi
In the central U.S. Corn Belt, corn and soybean production is mostly rainfed and highly relies on in-season weather conditions for successful crop production. Weather variability alone explains more than 75% of corn yield variation in several areas of this region. Rainfall and air temperature during the growing season play a significant role in spatial and temporal variation in corn and soybean yields, and therefore can be used to develop yield estimation models. These models provide opportunities to estimate crop yield prior to harvest, which can be beneficial for farmers to make informed decisions on marketing and post-harvest farm management.
In order to develop yield estimation models for corn and soybean, a study was conducted using weather data (May to August) and corn and soybean grain yields from 1990 to 2017. The study included 169 counties from agricultural districts in Minnesota, Iowa, Illinois, and Indiana (Fig. 1). Weekly, biweekly, and monthly average air temperature, diurnal air temperature difference (difference between daily maximum and minimum air temperatures), and total rainfall data at the county level were used with county-average annual corn and soybean grain yields for model development. Three types of statistical models were assessed, including multiple linear regression, general additive, and support vector machine (SVM) models.
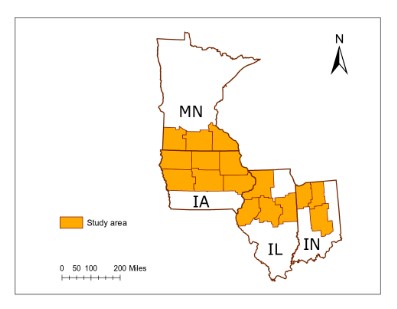
Fig. 1. States and their agricultural districts included
in this study.
Key findings
For both corn and soybean, the SVM model produced the most accurate estimates of county-average yields. For corn yield estimation, rainfall during June and July, along with average air temperature and diurnal air temperature difference during July and August were most important. Corn yield was generally higher when there was greater rainfall in June and July (corresponding with the vegetative to early reproductive stages), lower average air temperature in July and August (corresponding with the early to mid-reproductive stages), and greater diurnal air temperature difference in July and August.
For soybean, average air temperature in June, along with rainfall and diurnal air temperature difference in August were most important for yield estimation. Overall, soybean yield was greater when there was higher average air temperature in June (corresponding with the early to mid-vegetative stages) and greater rainfall and diurnal air temperature difference in August (corresponding with the early to mid- reproductive stages).
Lower diurnal air temperature difference occurs when there are warm nights, thereby increasing photorespiration in soybean and to a lesser degree in corn. Photorespiration reduces the quantity of photosynthetically derived sugars translocated to grain. Lower diurnal air temperature difference also decreases the duration of the reproductive stages in corn and soybean.
This study shows the usefulness of in-season weather data for reliable estimation of corn and soybean yields. It also indicates the importance of adequate soil moisture near the early reproductive stages, cool nights during the grain-filling period, and an extended duration of grain fill for high corn and soybean yields. Thus, although corn and soybean planting was delayed and crop development is behind normal this year, the outlook for yield is promising for corn and soybean fields with adequate stand establishment, particularly if weather conditions later in the growing season are favorable for crop development.
Source: umn.edu